Consensus Credibility Scores: a comprehensive dataset of Web domains’ credibility
Summary
Examining a source’s track record and editorial practices can be a useful guide in gauging the credibility with which to regard the content they publish and consequently the appropriate use. This approach has been used by various groups, including scientists, media professionals, and communities dedicated to skeptical inquiry, such as Wikipedia. Their work resulted in the compilation of lists of sources, predominantly web domains, to assist in research or to enable their communities to differentiate between sources deemed more or less trustworthy.
Science Feedback has identified dozens of these lists, created by “raters” with adequate expertise and compiled a comprehensive list of domains rated for their credibility. The final dataset comprises 24,000 domains across more than 100 countries, each associated with a score reflecting assessments of its credibility.
The aim is to help those, such as scientists and fact-checkers, who could need such a list for projects advancing the public interest.
Data Sources
The domain-level reliability ratings are derived from the data gathered using pre-existing, publicly-available evaluations from a broad range of expert raters, including academic researchers, media professionals and civil society organizations. This approach was inspired in significant part by the work of Lin et al (2023).
Aggregating data from many sources allows for:
- Maximized coverage: most raters focus on specific topics or geographies. Bringing together different raters allows for maximal coverage.
- Minimized bias: many popular domains are assessed independently by multiple raters, each bringing their own insight and method to evaluate the domain’s credibility. Using these multiple signals as input reduces the potential bias inherent to using any single rating methodology.
Criteria for dataset inclusion
These Consensus Credibility Scores are built by aggregating pre-existing lists of domain or URL credibility assessments published by media and information environment experts.
To be eligible for inclusion, the list must meet at least three of the five following criteria:
- A transparent, uniform rating methodology,
- Transparent reasoning justifying each rating,
- Be published by identifiable individuals or organizations recognized as subject matter experts,
- Be used in other reputable sources (peer-reviewed research, newspapers of record, scientific institutions…),
- Stem from an explicit commitment to non-partisanship, with no available evidence that the rater did not abide by this commitment.
These criteria were selected as they allowed, in our assessment, to meet high standards on the quality of the datasets while being sufficiently flexible to accommodate different types of raters and rating methods.
The number of domains rated is not a factor: the smallest list comprises 13 domains, while the largest covers 11,519 domains.
If you know of (or publish) another list that meets these criteria, please contact us.
Description of datasets
Science Feedback’s ratings use two main types of inputs: domain-level assessments of credibility and URL-level assessments of credibility.
Domain-level assessments datasets
Publishers of domain-level credibility ratings range widely, from academics to citizen projects to civil society researchers. The sources of domain-level credibility ratings we used are:
- 2023 Academic Ranking of World Universities
- Ad Fontes Media
- Bufale.net
- Bufalopedia
- Butac.it
- Center for Media, Data and Society series of reports on disinformation (1, 2, 3)
- Conspiracy Watch
- Courrier International
- European Media Systems Survey
- Grinberg et al (2019)
- IFCN
- VSquare’s ‘Firehose of Falsehood’ report
- Wikipedia blocked domains – France
- Wikipedia list of fake news websites – Philippines
- Wikipedia list of fake news websites in English
- Wikipedia discussions of website reliability in English
- Wikipedia discussions of website reliability in French
- Wikipedia discussions of website reliability in Swedish
- Wikipedia new page patrol
See technical appendix for more details.
URL-level assessments datasets
A number of organizations, including fact-checkers, primarily rate the credibility of individual pieces of content, such as specific articles on news websites or social media posts, without assigning an overall credibility grade to the source that published the content.
The sources of URL-level credibility ratings we used are:
See technical appendix for more details.
How ratings are calculated – Methodological overview
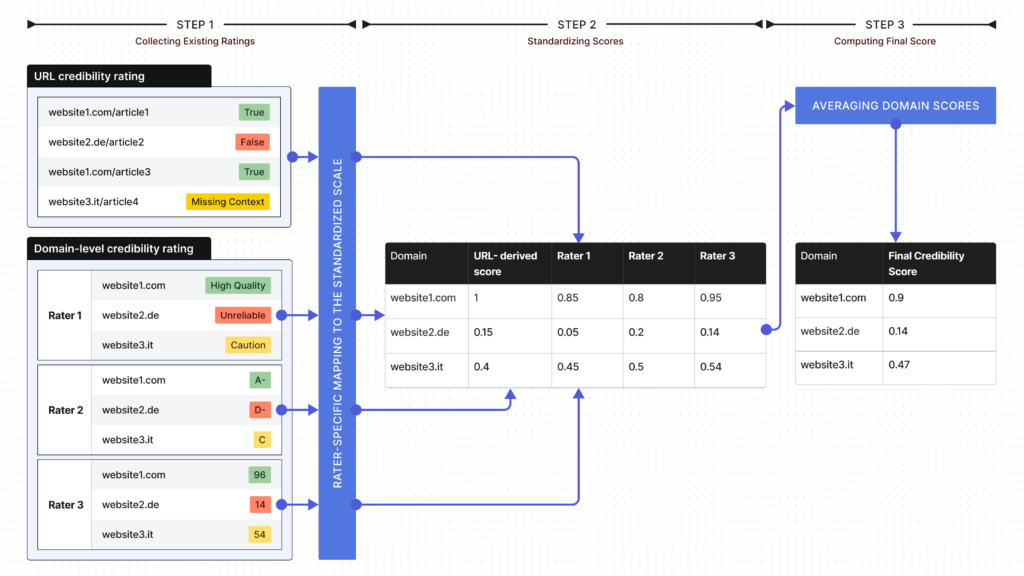
Mapping onto a common scale
A- Domain-level ratings
Some existing lists of domain credibility focus exclusively on identifying low-reliability websites, some focus on high-reliability ones, while others cover the full spectrum of reliability.
In addition to covering different spaces in the credibility spectrum, each rater uses its own rating scale. We have identified four main types of scales:
- Binary: the rater publishes a list of reliable/unreliable websites. Either a domain is in the list, or it isn’t.
- Category: the rater has a number of different categories (e.g. black list, grey list, white list or letter grades), which cover the full spectrum of reliability, assigning each domain to a discrete credibility bucket.
- Score: the rater has a continuous numeric scale which covers the full spectrum of reliability.
- Text: the rater does not provide an explicit grade, but it provides some textual assessment to assess the reliability of the domain.
In order to aggregate the lists into a single score, an intermediate step of conversion onto a common scale was conducted. The scale ranged from zero (lowest credibility) to one (highest credibility).
A source-specific mapping scale was developed for each rater (see the technical appendix for full details), building on the following general guidelines:
- When the original rating covered only one type of domain credibility (e.g. list of reliable domains), the same rating was assigned to all domains on the list.
The rating assigned was dependent on the rater’s own description of what type of websites make it into its list. For instance, bufale.net presents its collection as a ‘black list’. Consequently, all websites identified by bufale.net received a score of 0.
To the contrary, all academic institutions listed in the 2023 Academic Ranking of World Universities (also known as the Shanghai Ranking) received the maximum score of 1, under the assumption that the world’s leading universities publish credible information. - When the rater used a continuous numeric scale covering the full spectrum of information credibility, we either:
- Projected linearly the scores onto the zero-to-one scale,
- Used a quantile approach if a linear projection would have resulted in mostly-undifferentiated scores (for instance, if the list has a few outliers that compress the vast majority of the other ratings into a narrow range).
- When the rater used categories, the score assigned to each category depended on the rater’s own description of the categories.
If categories covered the full spectrum at (e.g. ‘Very low’, ’Low’, ’Medium’, ’High’, ’Very high’), a score of 0 was assigned to the lowest credibility category, a score of 1 to the highest, and the score of the intermediary categories linearly interpolated.
Otherwise, a human assessor assigned a score to each category based on the category’s description by the rater.
For instance, Raskrikavanje publishes both a ‘Red Flag’ and a ‘High Risk’ list of media. The ‘Red Flag’ list is described as ‘media that publish fake news’ while the ‘High Risk’ one contains ‘media that publish reports of questionable truthfulness’. Consequently, we assigned a score of 0 (lowest credibility) to websites on the Red Flag list, and 0.25 to websites on the High Risk list. - When the rater used natural language to describe domains’ credibility, a human annotator translated these assessments into a score between 0 and 1. Calibration was conducted on the basis of a baseline (does this rater include more reliable sources than unreliable ones or vice-versa?), and by looking at examples of the language used to describe high- and low-quality domains.
B- URL-level ratings
Fact-checkers, academics and expert civil society organizations also rate the credibility of individual pieces of content that circulate on the Internet. Although they are not directly domain ratings, these URL-level assessments contain useful information as to the credibility of the website to which they belong.
To build a domain-level score from these individual URL credibility assessments, a score was computed for each domain on the basis of the number of individual URLs belonging to that website that have been rated: each URL found to be carrying misinformation resulted in a ‘penalty’, while each URL found to be providing credible information resulted in a ‘reward’.
To account for website popularity (regardless of the strictness of their editorial standards, popular websites tend to be subject to more scrutiny than others), we normalize this score by dividing it by the number of monthly visitors to the website.
This normalized score is then projected onto the common scale using the mapping key detailed in the technical appendix.
Aggregating the scores
The reliability scores given to each domain are then averaged across raters to derive a final credibility score for the domain.
Further exploration of the most appropriate aggregating method could be conducted in future work. In particular, assigning different weights to different raters on the basis of their typical distance-to-consensus could be explored (on the assumption that raters that are consistently far from other raters should be given less weight than those that tend to fall close to the consensus).
Results
The resulting list of Consensus Credibility Scores covers 24,118 domains. This list aims to support public-interest projects; we therefore encourage all parties interested in it to reach out.
For the vast majority of use cases, Science Feedback recommends to group domains into credibility categories, and work using these categories rather than using individual scores. Our general recommendation is to slice the dataset as follows:
- Most reliable: 0.8 <= Consensus Credibility Score <= 1
- Generally reliable: 0.6 <= Consensus Credibility Score < 0.8
- Limited reliability: 0.4 <= Consensus Credibility Score < 0.6
- Generally unreliable: 0.2 <= Consensus Credibility Score < 0.4
- Least reliable: 0 <= Consensus Credibility Score < 0.2
Note that this specific categorization should be interpreted as a general guideline, different use cases might require different stratification.
Database sample
The full list with over 24,000 domain ratings is available free-of-charge for projects advancing the public interest: get in touch.
Geographic breakdown of origin of traffic
Leading geographic origin of traffic as estimated by Similarweb
Distribution of domain credibility scores
Acknowledgements
These domain ratings were developed as part of a project supported by the EMIF.
The sole responsibility for any content supported by the European Media and Information Fund lies with the author(s) and it may not necessarily reflect the positions of the EMIF and the Fund Partners, the Calouste Gulbenkian Foundation and the European University Institute.