- Energy
Training and using ChatGPT uses a lot of energy, but exact numbers are tricky to pin down without data from OpenAI
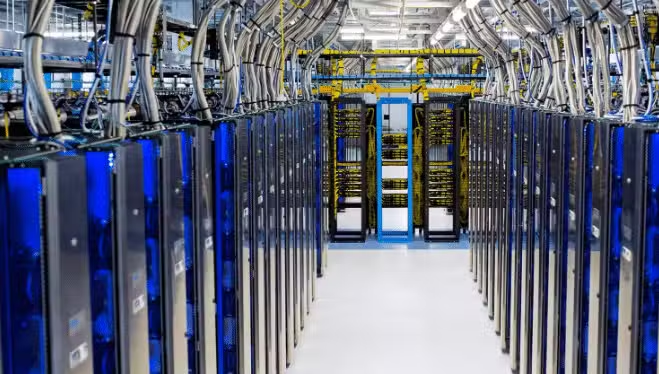
As the capabilities and adoption of artificial intelligence (AI) are rapidly growing, people have started scrutinizing its environmental costs. Across social media, users have made claims about the energy requirements of AI chatbots (like ChatGPT), often by comparing it to other activities or things in our daily lives.
For example, a Linkedin post from 15 February 2025 claimed that ‘using ChatGPT-4 10 times per day for one year emits the same amount of CO2 as taking one flight from New York to Paris’ (translated from French, here). The original source of this information appears to be an article on Vert – an independent French news outlet – from 21 November 2024.
Below we will investigate this claim and dig into some of the knowns and unknowns about energy consumption of AI chatbots like ChatGPT.
Main Takeaways:
As the capabilities and adoption of artificial intelligence (AI) continue to grow, so are its energy impacts. Training and ongoing use of AI models requires significant energy due to their use of data centers – one of the most energy-intensive building types.
Although this energy use is broadly understood, OpenAI has not released enough data for people to know exactly how much energy consumed when using their AI chatbots (like GPT-4). An online tool called ‘EcoLogits’ can estimate the energy consumption and CO2 emissions from using ChatGPT, but has limited precision given the lack of data from OpenAI.
The EcoLogits tool calculates emissions from multiple steps – raw material extraction, transport, usage, and end-of-life. Although that is a valid method, it can be misleading to compare these results to flight emissions (as was done in a recent social media post) which are more direct – fuel is burned, CO2 is emitted. That excludes other emissions steps required for a plane to fly, such as building the plane and transporting fuel, which would increase the carbon footprint if included.
Significant amounts of energy are needed for both training and ongoing use of ChatGPT – AI energy consumption is expected to grow
Before digging into the details: why investigate the energy use of ChatGPT in the first place? In short, both training and using AI models consumes significant amounts of energy by using data centers – one of the most energy-intensive building types, according to the U.S. Department of Energy. And adoption of these technologies has happened quite rapidly – in fact, according to the International Energy Agency (IEA), when comparing the percentage of households that adopted technologies after their commercial release, the adoption rate for generative AI has outpaced the rates for both personal computers and the internet (Figure 1).
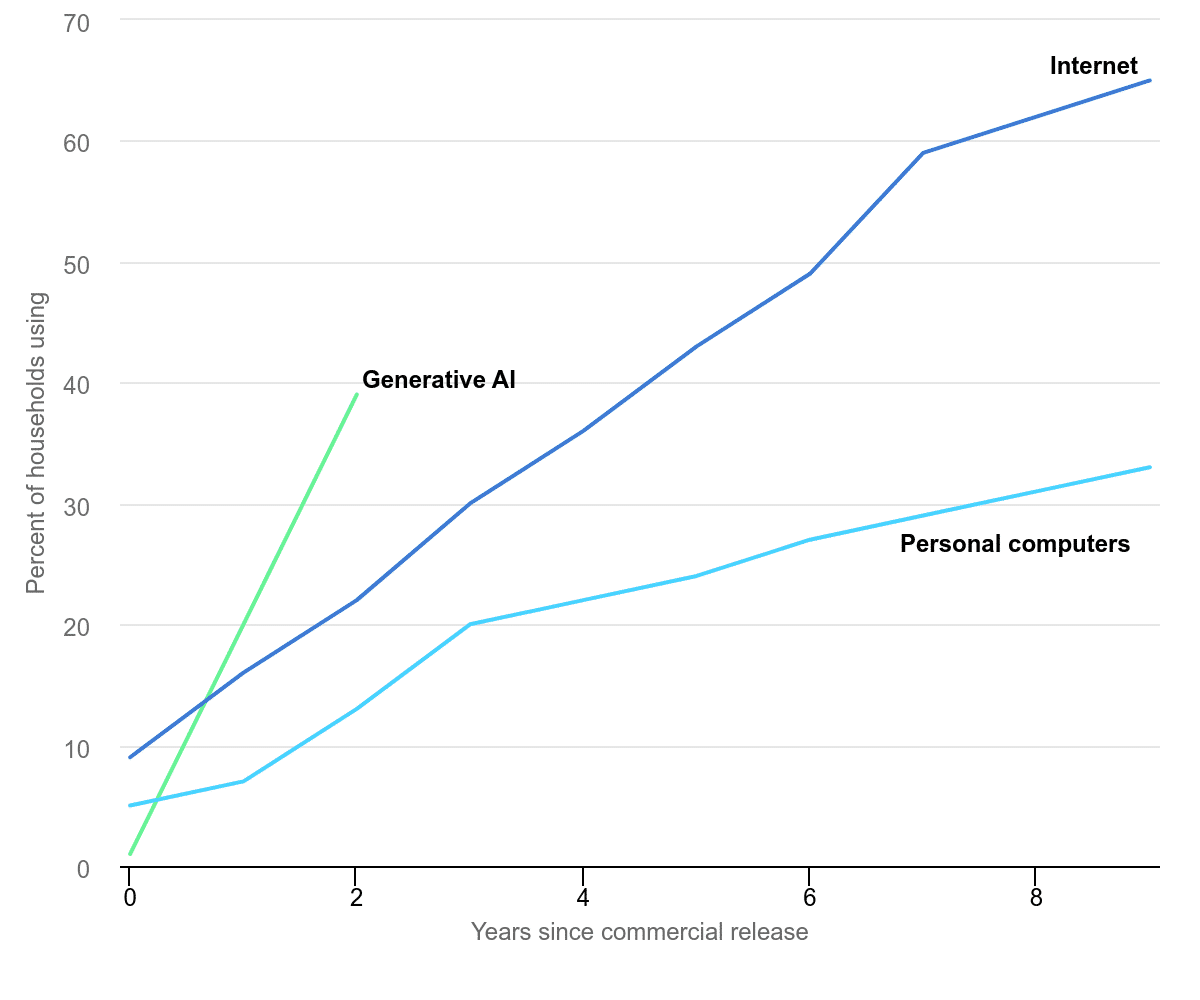
So what are the energy implications of this? Although it’s an ongoing topic of research, the IEA explains, “Electricity consumption from data centres, artificial intelligence (AI) and the cryptocurrency sector could double by 2026” – this combined electricity consumption would roughly match that of the entire country of Japan. But what about AI specifically?
As the number of users and capabilities of AI chatbots are growing, so are concerns about energy use. This was highlighted by Vijay Gadepally, senior scientist and principal investigator at the Massachusetts Institute of Technology (MIT) Lincoln Laboratory, in a January 2025 article published by MIT Sloan School of Management:
“As we move from text to video to image, these AI models are growing larger and larger, and so is their energy impact […] This is going to grow into a pretty sizable amount of energy use and a growing contributor to emissions across the world.”
At a broad scale, it is clear that there are energy and environmental impacts associated with the advancement and use of AI. But do we know enough to calculate the exact energy used or carbon dioxide (CO2) emitted per query/request to ChatGPT – or GPT-4 more specifically? At this moment, it’s quite tricky.
Numerous social media posts have discussed the energy that is used each time you send a query to ChatGPT, but it appears that few cite any sources for this information. And there may be a good reason for this: there appears to be a scarcity of data both from the company that developed the model (OpenAI) and in the scientific literature.
As a result, some have attempted to estimate this themselves using approximate values. For example, the claim we mentioned earlier – comparing hypothetical CO2 emissions between daily GPT-4 use and one transatlantic flight – used a tool called the ‘EcoLogits Calculator’ to do their estimations. The Linkedin post making this claim pulled information from a Vert article published on 21 November 2024, which detailed some underlying assumptions.
To check if this was an accurate comparison, Science Feedback sought to answer a few questions: did they use sound methodology, can we replicate their work to get the same values, and are there any uncertainties? We will investigate these below.
Emissions per query made to ChatGPT can only be roughly estimated due to large data gaps
Before we investigate, it’s worth noting that the Linkedin post did add a caveat that their calculations were ‘imperfect’ given the lack of data from OpenAI on this subject. However, these caveats can easily be forgotten or left out when people decide to share the simplified claim comparing ChatGPT use to a transatlantic flight.
So if people only hear or read the claim itself, would it be accurate on its own? As we will detail below, there are several reasons the claim alone could be misleading.
To start the investigation, Science Feedback first attempted to replicate the values in the ‘EcoLogits’ tool used to calculate greenhouse gas emissions and energy consumption. By following the assumptions laid out in the Vert article, Science Feedback arrived at the same values as shared in the article (Figure 2). However, the question still remains – how close are these values to reality?
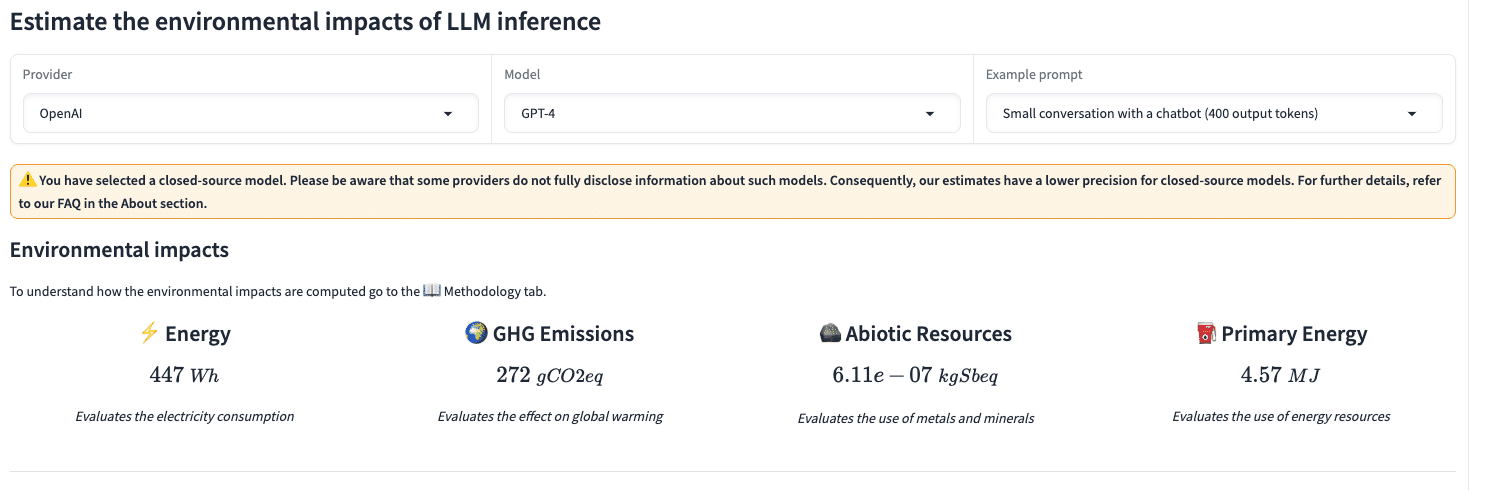
In fact, a note in the tool itself explains that by selecting a closed-source model like GPT-4, EcoLogits’ estimates will have a lower precision – confirming the issue we noted earlier about data scarcity. To gain more insights about this, Science Feedback reached out to Dr. Anne-Laure Ligozat, computer science professor at ENSIIE and LISN, who researches the environmental impacts of digital technology.
“The Ecologits tool used here is based on a sound methodology, but as you pointed out, the necessary data is not always available so the computation of the impacts requires to do a few hypotheses and approximations. The order of magnitude obtained is consistent with scientific publications [linked here and here, for example]”, Ligozat explains.
However, despite the order of magnitude of these values being consistent with other research – meaning the values aren’t 10 times greater or smaller – there are still uncertainties in these values. Ligozat explains, “The uncertainty is high because of these approximations, but I do not think uncertainty values are available for this tool.”
When asked about the accuracy and uncertainties involved in the claim specifically (i.e., the transatlantic flight comparison), Ligozat explained, “It probably gives a correct order of magnitude but multiplying the impacts for one inference may not be the best way to estimate the overall impacts in particular because it hides threshold effects.”
For those less familiar with those terms, let’s quickly unpack them. An inference is the process of a user sending a query to an trained AI model, which then applies its learning to find an answer for the user (Figure 3).
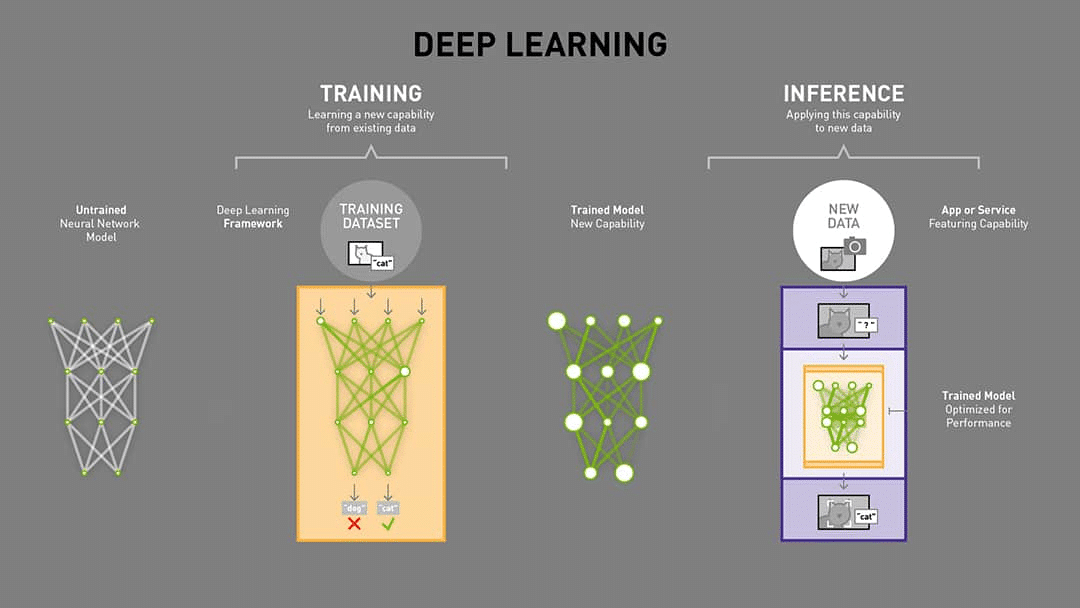
So in the quote above, Ligozat is pointing out that the claim may be oversimplifying a more complex scenario by using the EcoLogits tool to calculate emissions and energy use from one inference (query) and multiplying it (e.g., 10 times per day times 365 days per year). In other words, it isn’t necessarily the case that a one-off emissions scenario (like a single conversation on ChatGPT) can just be scaled as you’d wish by multiplying it. As Ligozat explains:
“The impact from executing several inferences may not be a multiple of executing one: for example, if you only have to process a few inferences a day, a small basic server may be enough, while if you have thousands a day, it will require a computation server with different characteristics.
I am not sure whether it would under- or over-estimate the impacts since the changes may go both ways: for example the manufacturing impacts of a bigger server may be significantly higher (increasing the footprint), but it may [process] one inference much more efficiently (decreasing the footprint).”
Between a lack of data released from OpenAI, the resulting lower precision in the EcoLogits estimates, and the method the Linkedin post used of ‘multiplying’ the effects across days and years, it seems that several layers of uncertainty are baked into the claim comparing ChatGPT queries to flight emissions.
It is also worth noting the method of calculating CO2 emissions per query/inference in the EcoLogits tool is based on a life cycle analysis methodology – not just direct emissions resulting from the energy consumed by using ChatGPT. In other words, while flight emissions are directly looking at the CO2 emitted per gallon of fossil fuel used, the EcoLogits tool methodology looks more broadly at the CO2 emitted from multiple steps of the process – extraction of raw materials, transport, usage, and end-of-life.
Science Feedback also found variation in CO2 emission calculations for a flight from New York to Paris using five different online calculators for flight-CO2 emissions. The values ranged from 322 kg of CO2 to 1000 kg of CO2 (roughly one tonne). However, the calculator with the most transparent and detailed methodology came from the International Civil Aviation Organization (ICAO) and gave a value of 322 kg of CO2 (Figure 4).
Notably, this is roughly one third of the CO2 emissions coming from the ‘10 times per day’ ChatGPT estimates. But, again, the ICAO methodology explains this is only CO2 from burning fuel – not an entire lifecycle analysis (as is performed in the EcoLogits estimates).
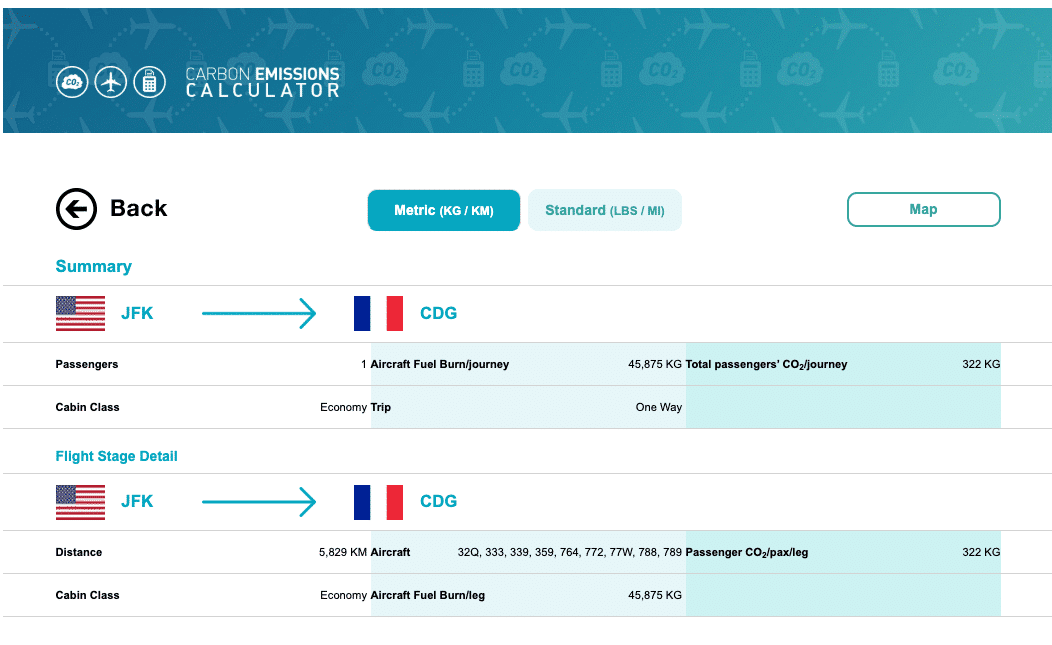
The comparison made in the claim from Linkedin may also be misleading because it does not take into account the range of complexity for each type of inference. The example from the claim is a ‘small’ conversation, but that seems to be chosen arbitrarily among other use cases.
For example, if a user wants to write a tweet instead, the CO2 emissions (per EcoLogits) for the same type of scenario (10 times per day for one year) drop from roughly 992 kg of CO2 per year down to 124 kg – a nearly 8-fold difference (Figure 5). In the ‘tweet’ example – using the EcoLogits calculator – the emissions would be roughly the same as one third of a flight from New York to Paris (depending on the flight-emissions calculator used).
In terms of energy consumption, the energy consumed by writing a single tweet with GPT-4 – according to EcoLogits estimates – could power a 60 watt incandescent light bulb (a standard household light bulb) for just under an hour. Note that energy in Figure 5 is given in ‘Wh’ or ‘watt-hours’, defined as the electrical power consumed by running something for one hour (hence a 60 watt bulb being able to run for just under an hour with 55 Wh of energy).
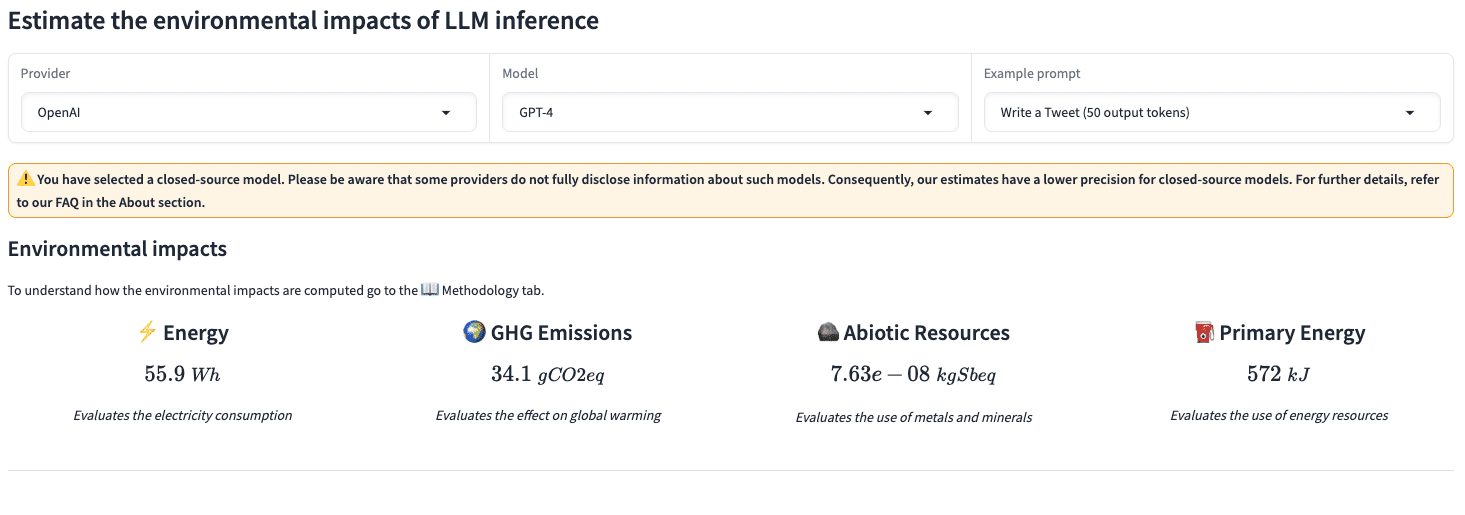
While these types of comparisons may help inform people of potential environmental impacts of using emerging technologies like AI, it may be a disservice to readers to cherry-pick examples while not explaining more context or being transparent about the uncertainties involved. Without that important context, people may notice wide discrepancies in estimates being shared on social media, devaluing a more important takeaway: AI is growing rapidly and is projected to further increase energy demands and greenhouse gas emissions.
Scientists’ Feedback
Questions from Science Feedback:
- Is there any way of approximating the energy consumption of an average GPT-4 query/search? Is there enough available data to do so?
- What level of uncertainty is involved in performing this type of energy calculation or approximation?
- Do you think that the claim above about CO2 emissions from using GPT-4 is an accurate approximation or are there too many data gaps or uncertainties to make such statements (i.e., comparing it to the emissions from taking a flight)?
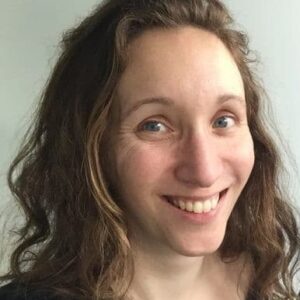
Anne-Laure Ligozat
Professor, LISN and ENSIIE
“1. The Ecologits tool used here is based on a sound methodology, but as you pointed out, the necessary data is not always available so the computation of the impacts requires to do a few hypotheses and approximations. The order of magnitude obtained is consistent with scientific publications [linked here and here, for example].
2. The uncertainty is high because of these approximations, but I do not think uncertainty values are available for this tool.
3. It probably gives a correct order of magnitude but multiplying the impacts for one inference may not be the best way to estimate the overall impacts in particular because it hides threshold effects. The impact from executing several inferences may not be a multiple of executing one: for example, if you only have to process a few inferences a day, a small basic server may be enough, while if you have thousands a day, it will require a computation server with different characteristics.
I am not sure whether it would under- or over-estimate the impacts since the changes may go both ways: for example the manufacturing impacts of a bigger server may be significantly higher (increasing the footprint), but it may [process] one inference much more efficiently (decreasing the footprint).”